AI Speech to Text Conversion
Admin / March 17, 2024
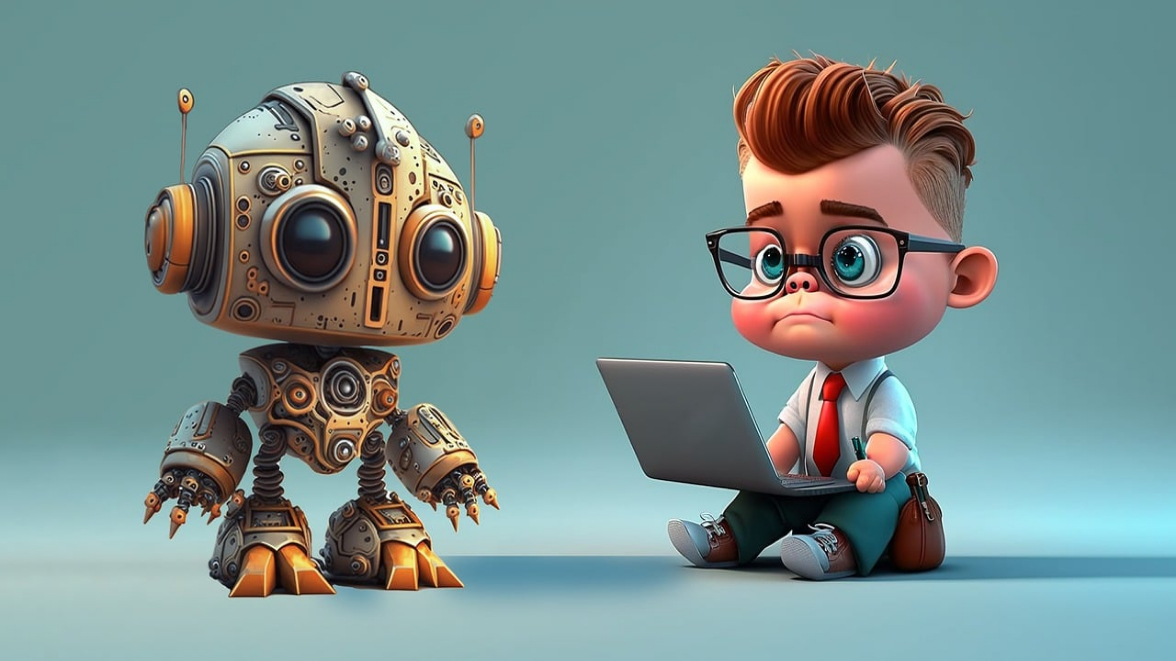
Challenges in AI Speech to Text Conversion
The accuracy and efficiency of AI speech to text conversion have been two significant challenges in the field. While AI technology has made remarkable progress in recent years, there are still limitations that need to be addressed to ensure reliable and seamless voice recognition and transcription.
One of the main challenges lies in the accuracy of AI speech to text conversion. Although AI systems have become quite proficient in recognizing speech, they still struggle with certain accents, dialects, and languages. Variations in pronunciation, intonation, and speech patterns can pose difficulties for AI algorithms, leading to inaccuracies in the transcribed text. This can be particularly problematic in scenarios where precise transcription is crucial, such as legal or medical documentation.
Another challenge is the efficiency of the conversion process. While AI algorithms can transcribe speech relatively quickly, there is room for improvement in terms of speed and real-time transcription. This is especially important in applications such as live captioning or transcription services, where immediate and accurate conversion is vital.
Innovations in AI technology for improved voice recognition and transcriptionIn
order to address the challenges in accuracy and efficiency, innovations in AI technology have been introduced to improve voice recognition and transcription capabilities.
One such innovation is the use of deep learning algorithms. Deep learning models, such as recurrent neural networks (RNNs) and convolutional neural networks (CNNs), have shown promising results in enhancing the accuracy of AI speech to text conversion. These models are capable of learning complex patterns and features from large amounts of training data, allowing them to better understand and interpret speech.
Furthermore, advancements in machine learning have enabled the development of more sophisticated language models. These models can capture the context and semantics of speech, making the transcription process more accurate and contextually aware. By incorporating contextual information, AI algorithms can better handle ambiguous or unclear speech, improving the overall accuracy of the conversion.
Improving Accuracy and Efficiency in AI Speech to Text Conversion
To improve the accuracy of AI speech to text conversion, deep learning techniques have been employed. Deep learning models can analyze speech patterns and identify subtle nuances in pronunciation, helping to overcome challenges posed by accents and dialects. By training these models on diverse datasets that encompass a wide range of speech variations, the accuracy of transcription can be significantly improved.
In addition to accuracy, efficiency in AI speech to text conversion can be enhanced through data optimization. By leveraging large-scale datasets and employing techniques such as data augmentation and transfer learning, AI algorithms can be trained to recognize speech more efficiently. This reduces the computational resources required for transcription, enabling faster and more real-time conversions.
Future Trends in AI Speech to Text ConversionAI speech to text conversion
is revolutionizing verbal communication, and future advancements in machine learning and deep learning are set to further improve its accuracy and capabilities.
Advancements in machine learning algorithms, such as transformer models, have shown great potential in capturing long-range dependencies and contextual information in speech. These models can better understand the flow and structure of conversations, leading to more accurate and coherent transcriptions.
Furthermore, as AI technology continues to evolve, we can expect improvements in the recognition of multiple speakers and the ability to differentiate between overlapping speech. This will be particularly useful in scenarios such as meetings or interviews, where multiple voices need to be transcribed accurately.
In conclusion, while challenges in accuracy and efficiency persist, innovations in AI technology are continuously improving the capabilities of speech to text conversion. With the integration of deep learning algorithms, advancements in machine learning, and the development of more sophisticated language models, AI speech to text conversion is becoming increasingly accurate and efficient. As these technologies continue to evolve, we can anticipate a future where verbal communication is seamlessly translated into written text, revolutionizing the way we interact and communicate.